- LLMs Research
- Posts
- LLMs related research papers published from September 9-13, 2024
LLMs related research papers published from September 9-13, 2024
From Accelerated Speech Recognition to Advanced Reasoning - weekly summary of papers improving LLMs
Dear readers,
This edition is long so you may need to click on “view entire content” button at the end to read full edition. Not my fault, it’s Google!
Speaking of Google, I have tried NotebookLM to create an interesting podcast discussing this edition’s research papers. If you don’t have time to read, LISTEN!😄
Share you thoughts if you liked this podcast format or want any modifications! This podcast is yours, treat it like one! - shower the comments for improvements.👏🏻
🔑 takeaway from today’s newsletter
AIPO refines LLMs to deliver concise and meaningful responses - enhancing alignment with human preferences for better AI interactions.
CPL introduces Critical Planning Step Learning, boosting LLM reasoning and significantly improving problem - solving accuracy.
Faster Speech-LLaMA accelerates speech recognition by predicting multiple tokens at once - reducing inference time without sacrificing accuracy.
AdaCAD dynamically balances contextual and parametric knowledge in LLMs, resolving conflicts for clearer and more accurate outputs.
E2LLM extends LLMs' capabilities to handle longer contexts efficiently - enhancing understanding and reasoning over extended inputs.
Core research improving LLMs!
Why?: Improving the alignment of LLMs through iterative preference optimization is important for their effective operation and scaling, as preference optimization is becoming a popular alternative to proximal policy optimization.
How?: AIPO introduces a new training method that encourages LLMs to produce concise and meaningful responses, addressing the length exploitation problem. It does so by:
Carefully selecting training data: AIPO focuses on using synthetic data, particularly instructions created by humans and responses generated by the LLM itself.
Iterative training: The model is trained in multiple rounds, incorporating feedback from a reference model (a more powerful LLM) in each round. This helps refine the model's responses over time.
Agreement-aware training: AIPO uses a special coefficient to adjust the training process based on how well the LLM's responses align with the reference model's preferences. This ensures that the LLM is rewarded for producing responses that are both concise and aligned with human-like preferences.
In simpler terms, imagine you're teaching a child to write good stories. Instead of just telling them to write longer stories, you:
Give them specific examples of good stories and let them learn from their own attempts.
Provide feedback in stages, gradually refining their writing style and content.
Reward them not just for length, but also for clarity, conciseness, and creativity, using a teacher's (reference model's) opinion as a guide.
AIPO applies a similar concept to LLMs, encouraging them to produce responses that are not just long, but also high-quality and aligned with human expectations.
Why?: Reasoning improvement of LLMs.
How?: This paper introduces Critical Planning Step Learning (CPL) that uses Monte Carlo Tree Search (MCTS) to identify diverse planning steps in multi-step reasoning tasks. CPL focuses on learning step-level planning preferences based on long-term outcomes to improve planning capabilities. It incorporates Step-level Advantage Preference Optimization (Step-APO), which integrates an advantage estimate for step-level preference pairs using MCTS within Direct Preference Optimization (DPO) techniques, capturing fine-grained supervision at each reasoning step. Experiments were conducted on GSM8K and MATH datasets.
Results: The CPL method significantly improved model performance on GSM8K +10.5% and MATH by 6.5%.
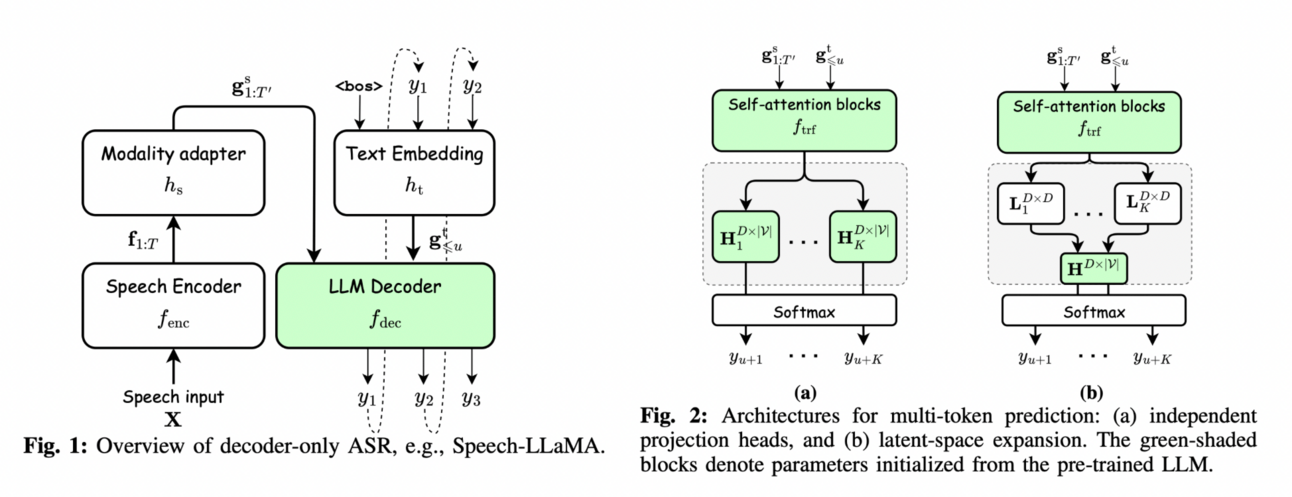
Why?: Reducing the inference time of Speech-LLaMA models.
How?: Meta team proposes predicting multiple tokens per decoding step instead of the traditional sequential approach. They explore various model architectures and implement threshold-based and verification-based inference strategies. Additionally, they introduce a prefix-based beam search decoding method for efficient minimum word error rate training. The models are then evaluated across several public speech recognition benchmarks.
Results: The proposed models achieve a 3.2x reduction in decoder calls while maintaining or improving word error rate (WER) performance.

Why?: The paper tries to resolve knowledge conflict in LLMs, where discrepancies between contextual and parametric information can impair performance.
How?: Paper introduces AdaCAD, a dynamic approach that calculates the degree of conflict between contextual and parametric knowledge using the Jensen-Shannon divergence. This calculated divergence helps to dynamically adjust the decoding process at an instance level, rather than using static methods. Tests involved comparing AdaCAD to other contrastive methods across four models on various QA and summarization tasks, focusing on how well it handles varying degrees of conflict.
Results: AdaCAD yielded an average accuracy gain of 14.21% over a static contrastive baseline.
This research paper argues that limiting human preferences to binary choices is an oversimplification and proposes using soft preference labels, represented by a probability (p̂), to reflect the nuanced nature of human preferences. To incorporate these soft labels into LLMs alignment, the authors introduce weighted geometric averaging into the loss function of Direct Preference Optimization (DPO) and related algorithms like IPO and ROPO. This method weights the likelihoods of the chosen and rejected responses based on the soft preference label—strong preferences (p̂ closer to 1) result in larger gradient updates during training than weak preferences (p̂ closer to 0.5). This weighting scheme allows the model to prioritize learning from high-confidence preference pairs while mitigating the effects of noisy data points and the tendency to over-optimize for response length.

Why?: Extends context length
How?: Paper introduces E2LLM, a model that enhances long-context capabilities by segmenting long inputs into chunks, which are compressed into embedding vectors using a pre-trained text encoder. These embeddings are then aligned with a decoder-only LLM through an adapter. The training objectives focus on reconstructing the encoder’s output and fine-tuning for long-context instructions, aiding LLMs in interpreting soft prompts.
Why?: Current LLMs which are trained with Direct Preference Optimization (DPO) suffer from excessive verbosity.
How?: Paper performs a theoretical analysis of DPO and its tendency to correlate rewards with data length, leading to verbosity.
To handle this, paper introduces LD-DPO, a novel algorithm designed to mitigate length sensitivity in DPO by decoupling the preference for verbosity from other human-like preferences.
LD-DPO works by:
Identifying the "public length" (lp) of a response pair—the number of tokens shared by both responses.
Decomposing the likelihood of the longer response into the product of the likelihood of the public-length portion and the likelihood of the "excessive" portion.
Introducing a hyperparameter α (ranging from 0 to 1) to control the influence of the excessive portion on the likelihood. A lower α weakens the impact of the excessive length, reducing DPO's sensitivity to it.
By adjusting α, LD-DPO aims to find a balance between reducing verbosity and preserving the LLM's ability to learn other valuable preferences from the training data.
Results: LD-DPO consistently reduced response lengths by 10-40% compared to DPO.
Why?: Paper explores the inner workings of LLMs beyond token-level predictions.
How?: Paper analyze the role of the "\n\n" double newline token in single-token activations. The authors explore how these activations encode information about the content of subsequent paragraphs. They employ a method of patching these activations to assess their information transfer capabilities regarding contextual understanding in paragraph formation.
Why?: This research claims that hallucination is not a bug in LLMs that can be solved instead it is a nature of LLM and is inevitable.
How?: The paper uses mathematical concepts and logic to argue that several factors make structural hallucinations inevitable:
Incomplete Training Data: It's impossible to capture all of human knowledge in a dataset, even a massive one. This incompleteness means that LLMs will always encounter situations or facts they haven't seen before, leading to potential hallucinations.
Difficulty Finding the Right Information: Even with a complete dataset, finding the exact information needed for a specific query can be extremely difficult for an LLM. Think of it like searching for a needle in a haystack—there's just too much data to sift through perfectly.
Misinterpreting What We Mean: Natural language is ambiguous, meaning the same phrase can have different meanings depending on the context. LLMs often struggle to correctly interpret the true meaning behind our prompts, leading to hallucinations.
Unpredictable Outputs: The paper draws a parallel between how LLMs generate text and a famous problem in computer science called the "Halting Problem." Basically, it's impossible to perfectly predict what output an LLM will generate beforehand, just like it's impossible to always know if a computer program will run forever or eventually stop. This unpredictability makes hallucinations unavoidable.
Fact-Checking Isn't Foolproof: While fact-checking can help, the paper argues that it's mathematically impossible to have a fact-checking system that catches every single hallucination.
The Bottom Line: We need to accept that LLMs will always have the potential to hallucinate and focus on developing strategies to manage and mitigate this risk.
Why?: Paper improves reasoning ability in LLMs.
How?: The research introduces a neuro-symbolic methodology that integrates neuro-symbolic reasoning into the LLM training process. This involves developing a loss function based on logical consistency with a predefined set of facts and rules, allowing the LLM to be trained to adhere to these constraints. The approach is balanced between full fine-tuning and offloading reasoning tasks to external tools, aiming to teach LLMs logical consistency with minimal fine-tuning. It also facilitates the inclusion and integration of multiple logical constraints efficiently, enabling the models to handle a wide range of logical requirements.
LLMOps & GPU level optimization
Why?: The research manages LLMs inferencing workloads utilizing the partitioning feature, Multi-Instance GPU (MIG).
How?: This paper developed two approaches: an optimization method and a heuristic method. Both aim to efficiently place or migrate workloads to minimize the number of GPUs used and reduce memory and compute wastage.
Results: The research demonstrated up to a 2.85x improvement in the number of GPUs used and up to a 70% reduction in GPU wastage over baseline heuristics.
Why?: Deploying multimodal large language models (MLLMs) on edge devices.
How?: The paper introduce Inf-MLLM, a framework for efficient streaming inference of MLLMs on a single GPU. They identify a novel attention pattern called 'attention saddles' that enables the system to maintain a size-constrained key-value (KV) cache. The cache dynamically stores recent and relevant tokens, reducing memory usage. The framework also implements 'attention bias' to capture long-term dependencies. This approach facilitates the inference of texts up to 4 million tokens long in multi-turn conversations and long videos.
Results: Inf-MLLM achieves stable performance on texts with 4 million tokens and multi-round conversations, offering superior streaming reasoning quality and 2x speedup compared to H2O.
Why?: To address bandwidth limitations in LLM training.
How?: This paper introduced DFabric, a two-tier interconnect architecture that enhances data communication across multiple hosts. First, it disaggregates computing units with a CXL fabric at the rack level for efficient intra-rack communication. Second, it disaggregates NICs from hosts into a centralized NIC pool using CXL fabric to enhance communication across racks. To tackle local memory access bottlenecks, DFabric creates a memory pool by disaggregating and expanding host memory.
LLMs evaluations
Windows Agent Arena, a benchmark environment for LLMs operating in the Windows OS. It utilizes the OSWorld framework to create 150+ tasks that test agents' planning, screen understanding, and tool usage within real Windows applications. The benchmark is scalable and can be evaluated quickly using parallelized computing on Azure. A new multi-modal agent called Navi is also introduced to showcase the capabilities of the benchmark.
Survey papers
Let’s make LLMs safe!!
Why?: The research provides a way to protect the intellectual property of LLMs.
How?: The research introduces FP-VEC, a technique that creates a fingerprint vector representing a confidential signature embedded in the LLM. This method enables the integration of the fingerprint into an unlimited number of LLMs simply through vector addition. The approach is lightweight, allowing fingerprinting using CPU-only devices, and maintains the model's typical behavior while being scalable with a single training process.
Why?: The research propose a safety mechanism for the critical vulnerability of Large Vision-Language Models (LVLMs) against adversarial and jailbreak attacks, which can lead to misleading or harmful outputs.
How?: The research introduces Sim-CLIP+, a defense mechanism that uses a Siamese architecture to adversarially fine-tune the CLIP vision encoder. The method maximizes cosine similarity between perturbed and clean samples to enhance robustness against adversarial attacks. Sim-CLIP+ is designed as a plug-and-play solution without requiring structural changes to the existing LVLM frameworks and maintains minimal computational overhead.
Why?: Membership inference attacks can reveal whether specific data points were part of the training dataset, compromising user privacy.
How?: The researchers developed a novel approach to membership inference attacks tailored to LLMs by leveraging the perplexity dynamics of subsequences within a token sequence. This method departs from traditional loss-based attacks that are inadequate for LLMs. By focusing on context-dependent variations, the researchers adapt statistical tests to uncover how these models memorize and reveal training data.
Why?: The research makes models more reliable and honest without needing real-time interventions.
How?: The research identifies activation vectors related to honesty in Llama-2-13b-chat. These vectors are added to residual stream activations to control model honesty. The novelty lies in directly fine-tuning these vectors into the model through a dual loss function combining cosine similarity of activations and a standard token-based loss. This method called 'representation tuning,' is compared to traditional fine-tuning and online steering.
Results: Fine-tuning vectors using the dual loss function increased honesty more effectively than online steering and generalized better than using a standard token-based loss.
Paper develop an adaptive position pre-fill jailbreak attack approach that first uses the LLM to generate safe output content. Then, by exploiting the model's ability to follow instructions and shift narratives, the attack prompts the LLM to produce harmful content. The approach was evaluated through extensive black-box experiments, targeting a well-known secure model, Llama2, effectively aligning with the model's differential output-stage alignment protection capabilities.
Results: This method increased the attack success rate by 47% on Llama2.
Creative ways to use LLMs!!
LLaQo: Towards a Query-Based Coach in Expressive Music Performance Assessment - Providing feedback in music performance for music lessons

The research proposes LLaQo, a query-based coaching model that evaluates music performances by processing audio data to provide insightful feedback. The system uses instruction-tuned query-response datasets covering music aspects like pitch accuracy and technique. It utilizes AudioMAE encoder and Vicuna-7b as a backend to predict performance ratings, understand contextual aspects like piece difficulty, and answer open-ended questions. LLaQo was trained to align responses with teacher assessments, achieving SOTA in predicting performance ratings.
Large Language Model Can Transcribe Speech in Multi-Talker Scenarios with Versatile Instructions - LLMs in multi-talker speech transcription

The study utilizes WavLM and Whisper encoders to capture detailed speech representations that consider speaker-specific traits and the context. These encoded features are then input into the large language model. The LLM is fine-tuned using the Low-Rank Adaptation (LoRA) method, enhancing its ability to comprehend and transcribe speech amidst multiple speakers. The model is tailored to follow various instructions and respond to differences such as language, speaker characteristics, and keywords.
Enabling Cost-Effective UI Automation Testing with Retrieval-Based LLMs: A Case Study in WeChat - LLMs for UI automation

The researchers developed CAT, a system combining LLMs and machine learning. CAT uses Retrieval Augmented Generation (RAG) to gather contextual examples for few-shot learning, guiding LLMs in generating actions for UI tasks. These tasks are then mapped onto the UI using machine learning, with LLMs optimizing the process. CAT was tested on WeChat, showcasing its integration into the application’s existing testing platform.
Enhancing Long Video Understanding via Hierarchical Event-Based Memory - Long video understanding in LLMs

The researchers propose a Hierarchical Event-based Memory-enhanced LLM (HEM-LLM) to better handle long video understanding by developing an adaptive sequence segmentation scheme that divides long videos into separate events. Each event is then individually modeled to reduce redundancy and preserve key semantics. Information from a prior event is compressed and incorporated while modeling the current event to maintain inter-event dependencies over the long term. The approach aims to improve video comprehension by maintaining contextual connections within events and across the video as a whole.
The research proposes a decentralized, multi-agent framework for the control of particle accelerators, powered by LLMs. The framework envisions a system where autonomous agents are responsible for high-level tasks and communication, with each agent specializing in controlling individual accelerator components. The system allows for self-improvement through experience and human interaction. Two examples are provided to demonstrate the feasibility of this architecture, raising questions about future AI applications in this field and the implications of integrating human feedback into the system.
Google researchers introduces a method using LLMs to comprehend advertiser intent in relation to content policy violations. It constructs advertiser content profiles by aggregating signals from ads, domains, and targeting information and utilizes LLMs for classification. Additionally, LLMs leverage prior knowledge about advertisers to predict policy violations. Minimal prompt tuning was conducted to enhance model performance.
LLMs for robotics & VLLMs

Why?: The research enhance robot navigation by using human-like understanding of LLMs, allowing robots to utilize external and experiential information akin to humans.
How?: The study proposes osmAG, a semantic topometric hierarchical map format, to integrate LLMs into robot navigation systems. This involves using the LLM as a copilot, which allows for the assimilation of diverse informational inputs. The methodology bridges the contextual understanding of LLMs with the spatial capabilities of traditional systems like ROS move_base, enhancing the flexibility and adaptability of robotic navigation.

Why?: The deploys LLMs on resource-constrained embedded devices, improving their performance.
How?: The researchers designed an FPGA-based accelerator to enhance LLM inference on embedded devices. They used post-training quantization to reduce memory usage and optimized for off-chip memory bandwidth. The architecture features asynchronous computation and full pipelining for efficient matrix-vector multiplication. The experiments evaluated the accelerator using the TinyLlama 1.1B model on a Xilinx ZCU102 platform.
Results: Experiments demonstrated a 14.3-15.8x speedup and a 6.1x power efficiency improvement compared to running solely on the ZCU102 processing system.
Reply