- LLMs Research
- Posts
- LLMs related research papers published in November 2024
LLMs related research papers published in November 2024
Discussing Key Innovations and Breakthroughs Transforming Large Language Models (LLMs)

🔑 takeaway from today’s newsletter
Smarter Thinking: Fixing logic gaps with Critical Tokens and tackling multi-hop reasoning challenges.
Efficient Fine-Tuning: Innovations like LoRA-SB cut costs without compromising performance.
Compact Models: Faster, lighter LLMs with breakthroughs like FlexiBit and MixPE.
Creative Applications: Endless panoramas, AI storytelling, and dynamic simulations powered by LLMs.
Sharper Understanding: Syntax tools and self-distillation improve accuracy and versatility.
🔑 Fun & engaging podcast using NotebookLM
Don’t have much time to read entire newsletter? Well, listen to this fun and engaging podcast covering these research papers in a detailed manner!
Token-Level Reasoning and Syntax

Why?: Discriminating influential 'critical tokens' which leads to incorrect reasoning paths can help improve LLMs reasoning ability.
How?: The authors propose a method called cDPO (Contrastive Direct Preference Optimization) to identify and correct these troublesome tokens. The method is built on the insight that not all tokens are equally important; some can drastically shift the model’s reasoning trajectory.
Positive vs. Negative Reasoning Trajectories
They collect positive (correct) reasoning paths and negative (incorrect) ones, often from a chain-of-thought or step-by-step solution. The key is to figure out which tokens appeared in correct vs. incorrect solutions, and how likely the LLM is to generate those tokens under different conditions.
Contrasting Likelihoods
They train two separate LLM “heads” or fine-tuned variants: one favoring positive tokens, the other favoring negative tokens. This allows them to contrast how likely the model is to produce certain “critical tokens” in each scenario. If a token is much more probable in the negative model, it might be a strong predictor of failure.
Token-Level DPO
Direct Preference Optimization (DPO) is usually done at the sequence level (i.e., preferring one entire output over another). The authors adapt DPO at the token level—pinpointing precisely where the model’s reasoning diverges. This token-level alignment is the core innovation: rather than treating a whole reasoning chain as “good or bad,” they surgically intervene on the problematic tokens.

Why?: Paper addresses the need for syntactic inductive biases in transformer language models to improve their robustness and data efficiency, without limiting model expressivity or increasing inference complexity.
How?: The authors propose TreeReg, a technique that blends syntactic constraints into a Transformer’s hidden stateswithout altering the core model architecture. Rather than fully retraining a parser, they apply a carefully designed auxiliary loss that leverages syntactic bracketing (e.g., parse trees).
Syntactic Brackets
Traditional syntactic parsers produce bracketed structures indicating phrases, clauses, etc.
These brackets encode where one syntactic constituent ends and another begins.
Orthogonality Constraints
TreeReg translates bracket information into differentiable constraints on hidden states: if two tokens are in the same syntactic constituent, their vector representations should align differently than tokens in different constituents.
By encouraging orthogonality (or closeness) based on bracket positions, the model “feels” the syntactic boundaries directly in its feature space.
No Architecture Overhaul
Crucially, no new parameters or special modules are added. Instead, the model gains a syntax-aware training signal through a supplementary loss that can be combined with standard next-token prediction or classification losses.
Why?: Existing tokenization methods, such as Byte-Pair Encoding (BPE), which often obscure the internal character structures within tokens, hindering LLMs' ability to grasp these details and perform effectively on tasks with limited data.
How?: Paper introduces a method called Token Internal Position Awareness (TIPA), which trains LLMs on reverse character prediction tasks using the tokenizer's vocabulary. This approach helps models learn internal token structures and character positions, enhancing their understanding.
Results: LLMs trained with TIPA outperform baseline models in predicting character positions at the token level and show improved performance and faster convergence on the downstream task of Chinese Spelling Correction (CSC).
Why?: Understanding the limitations of LLMs in performing internal reasoning without explicit chain-of-thought helps to enhance their reasoning capabilities, which is essential for advancing LLMs applications.
How?: Paper introduces a controlled experimental setting to assess two-hop reasoning in LLMs by training models on fictional facts and testing them on their ability to perform reasoning tasks both with and without chain-of-thought (CoT) aid. The models, including Llama 3 8B Instruct and GPT-4o, were evaluated on their performance in generalizing and composing learned facts across different documents and prompts.
Results: Models succeeded at two-hop reasoning using CoT but failed when reasoning required internal inference without CoT. The failure was evident when learned facts were in separate documents, highlighting LLMs inability for latent multi-hop reasoning without external aids in over half of the cases.
Efficient and Effective Fine-Tuning
Initialization using Update Approximation is a Silver Bullet for Extremely Efficient Low-Rank Fine-Tuning - GitHub

Why?: Fine-tuning LLMs often involves updating billions of parameters, which is computationally expensive and requires high-end hardware. LoRA can drastically reduce the number of trainable parameters by decomposing the weight updates into low-rank matrices. However, standard LoRA approaches often lag behind full fine-tuning in terms of performance and may demand extensive hyperparameter tuning to close that gap.
How?: The authors observe that while LoRA significantly cuts parameter counts, its performance can be very sensitive to how the low-rank matrices are initialized and scaled. Traditional approaches typically rely on random or naive initializations, requiring lengthy hyperparameter sweeps.
Gradient-Based Approximation
LoRA-SB approximates the initial gradient of a full fine-tuning step to seed the low-rank matrices. By focusing on the directions in parameter space that matter most for the task, it effectively “zeroes in” on the relevant updates right from the start.
Constrained Update Space
The low-rank adapters operate in a restricted subspace compared to full model updates. Finding the best possible initialization in that subspace is critical because every parameter or gradient dimension counts more when you have fewer degrees of freedom.
No Extra Hyperparameters
A major advantage is that LoRA-SB does not introduce additional hyper parameters. It’s a straightforward re-initialization scheme that can be easily integrated into existing LoRA pipelines.
Results: LoRA-SB outperforms standard LoRA and LoRA-XS, achieving efficient fine-tuning with 27-90x fewer parameters while maintaining high performance across various reasoning and language tasks.

Why?: Deploying LLMs on resource-constrained hardware (e.g., a single GPU or edge devices) can be extremely expensive and slow at inference time.
Neural Architecture Search (NAS) can find specialized, smaller architectures—but typically requires extensive compute and big teacher models.
Knowledge distillation helps preserve performance in a smaller model, but it’s often done in a global, all-at-once manner that doesn’t account for hardware constraints at a granular level.
The Puzzle framework aims to co-optimize:
Architecture design (via NAS) and
Performance retention (via distillation),
all while ensuring the resulting model meets specific hardware constraints (e.g., fits on a single GPU with minimal latency).
How?: Puzzle combines blockwise local knowledge distillation (BLD) with mixed-integer programming to systematically prune and restructure an LLM, step by step, into a smaller yet high-performing network.
Blockwise Local Distillation (BLD)
Instead of training a smaller model from scratch or distilling globally all at once, the approach partitions the original model into “blocks.” Each block is distilled locally, ensuring the sub-architecture within that block preserves knowledge from the teacher. This local focus can make distillation more stable and more aligned with the final architecture changes.
Mixed-Integer Programming for NAS
Puzzle sets hardware constraints (e.g., model size, memory footprint, or inference time) as optimization objectives. It then systematically selects or prunes blocks and layers, guided by a global objective function. Mixed-integer programming finds an optimal combination of blocks that fit the constraints while aiming for maximal performance.
Iterative Refinement
The framework iterates, refining architecture choices and distilling block by block. Each iteration zeros in on a final architecture that best balances size, speed, and accuracy.
Results: Nemotron-51B, derived from Puzzle, achieves 2.17x inference speedup fitting on a single NVIDIA H100 GPU, retaining 98.4% of the teacher model’s performance.

How?: The researchers propose a model-agnostic self-distillation method, DynSDPB, which learns from the model's own previous mini-batch outputs. This approach dynamically adjusts distillation influence and temperature to improve early iteration accuracy. It is a fine-tuning policy that integrates self-correction and self-training methods without architectural modification.
Previous Mini-Batch Outputs: Instead of referencing an external teacher, the model looks at its own predictions (logits, soft labels) from the previous mini-batch. These predictions become the pseudo “teacher” for the next iteration.
Dynamic Adjustments: The distillation influence (how strongly the model trusts its past predictions) and temperature (softening or sharpening the pseudo labels) are adjusted on the fly during fine-tuning. This dynamic tuning ensures the self-distillation signal remains useful and stable as the model learns.
No Extra Architectural Changes: Importantly, no additional layers or model capacity is required—just a revised fine-tuning policy that leverages the previous batch’s logits.
A word from today’s sponsor!
Love Hacker News but don’t have the time to read it every day? Try TLDR’s free daily newsletter. TLDR covers the best tech, startup, and coding stories in a quick email that takes 5 minutes to read. No politics, sports, or weather (we promise). And it's read by over 1,250,000 people!
Subscribe for free now and you'll get our next newsletter tomorrow morning.
Quantization: Memory & Speed Gains

Why?: Paper explores how low-bit quantization interacts with the training level of LLMs, uncovering implications for model efficiency and future training strategies.
How?: The study involved analyzing over 1500 quantized LLM checkpoints with variations in model size and training levels. Scaling laws were derived to explore the relationship between quantization-induced degradation (QiD) and factors like training tokens, model size, and bit width.
Results: The study revealed that undertrained models are less susceptible to QiD impacts compared to fully trained small models. These insights provide benchmarks and predictions for quantization performance in massive future models expected to be trained with 100 trillion tokens.
Why?: Quantization often impacts performance unevenly across layers, but identifying which layers tolerate fewer bits while maintaining overall model quality is a challenge. This research introduces a systematic framework to minimize this trade-off, optimizing layer-specific quantization.
How?: The authors introduce the 'linearity theorem' to correlate layer-wise reconstruction error with increased perplexity due to quantization. They developed the HIGGS method employing Hadamard rotations and MSE-optimal grids and proposed an efficient dynamic programming approach for non-uniform per-layer quantization.
Results: The proposed methods enhance accuracy-compression trade-offs for Llama-3.1, Llama-3.2, and Qwen models, outperforming existing data-free approaches like NF4.

Why?: The research tackles the computational and memory challenges inherent in deploying LLMs by introducing a more efficient quantization approach.
How?: MixPE introduces a mixed-precision processing element to enhance inference efficiency. It minimizes dequantization operations using two innovations: performing dequantization post per-group mixed-precision matrix multiplication and replacing traditional multipliers with shift add operations, thus boosting computational and energy efficiency.
Results: MixPE achieves a 2.6× speedup and 1.4× energy reduction over current quantization accelerators.

Why?: Current hardware accelerators are rigid, often supporting only standard precisions (e.g., FP16 or INT8). This limitation hampers the potential of custom mixed-precision arithmetic, which could better exploit model-specific needs and improve performance.
How?: FlexiBit proposes FlexiBit, a bit-parallel accelerator architecture which supports flexible precision and dynamic formats. It overcomes the limitations of bit-serial designs by enabling arbitrary mixed-precision computations, allowing models to adapt precision based on layer or task-specific requirements.
Results: FlexiBit achieved 1.66x to 3.9x higher performance per area compared to existing architectures on GPT-3 using FP6 precision.
Infrastructure, Caching, and Serving Optimizations
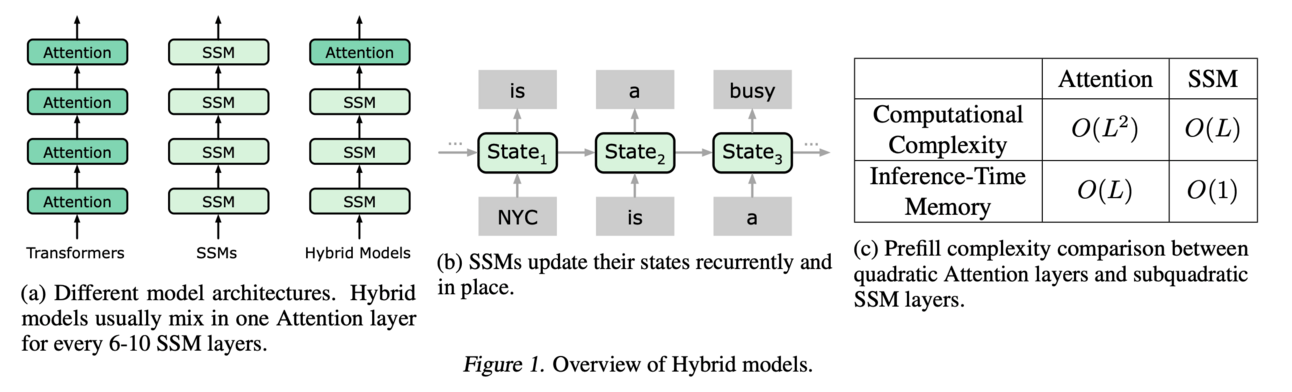
Why?: Hybrid LLMs, which combine attention and recurrent layers, often waste compute and memory when caching prefixes. Traditional caching systems rely on exact-match caching, which is inefficient for hybrid models that process partially overlapping prefixes.
How?: Marconi introduces novel cache admission and eviction policies that evaluate potential entries based on recency, predicted reuse likelihood, and computational savings versus memory costs. This approach allows efficient prefix caching for hybrid models, correcting traditional systems that require exact-match caches.
Results: Marconi achieves up to 34.4× higher token hit rates and reduces time-to-first-token by 617 ms compared to existing systems, indicating significant performance improvements.

Why?: The KV (key-value) cache is a critical bottleneck in long-context LLM inference due to its high memory requirements. Standard 8-bit compression methods are insufficient for scaling to larger models and contexts.
How?: The study introduces MiniKV, a KV cache optimization method using a novel 2-bit layer-discriminative approach, preserving accuracy while significantly reducing cache size. Specialized CUDA kernels were developed to ensure compatibility with FlashAttention, tested across various long-context tasks.
Results: Experiments demonstrated reduction in KV cache size by 86% with minimal accuracy loss.
Why?: In multi-user LLM-serving systems, inefficient context switching leads to fairness issues, where certain users face delays due to resource contention or idling GPUs. Improving context-switching efficiency ensures fairer service-level objectives (SLOs) for all users.
How?: The researchers developed FastSwitch, a system that maintains memory allocation while reducing context-switching overhead through enhancements like better I/O utilization and minimizing GPU idleness, responding to identified inefficiencies in current systems.
Results: FastSwitch demonstrated significant performance improvements over existing systems like vLLM, with speedups ranging from 1.4 to 11.2 times in various metrics.

How?: Paper proposes InstCache, which predicts user instructions using an instruction-aligned LLM. They implement an instruction pre-population algorithm based on the negative log likelihood to optimize cache size and hit rate. InstCache is executed as a hash table to minimize lookup latency, allowing for quick deployment.
Results: InstCache attains a 51.3% improvement in cache hit rate.
Why?: The computational cost of test-time inference grows with model size and sequence length, often creating bottlenecks in scalable deployments. Reducing test-time compute without sacrificing accuracy is crucial for scaling LLMs efficiently.
How?: Paper proposes a two-stage algorithm: (1) Generate N candidate solutions using the LLM. (2) Select the best solution via a K-round knockout tournament that compares pairs of solutions.
The algorithm leverages parallelization and requires only N × (K + 1) LLM calls.
Hallucination and attribution
Why?: Understanding how context influences LLM behavior is crucial for improving interpretability and efficiency. However, calculating context attribution is computationally expensive.
How?: Paper introduces AttriBoT, using cached activations to avoid redundant operations, hierarchical attribution for reduced computation, and proxy models to emulate the behavior of larger models. This approach approximates the LOO error more efficiently than previous methods.
Results: AttriBoT achieves over 300x speedup in computing context attributions, making it 30x faster than generating the response, while maintaining fidelity to target model's LOO error.

Why?: Training dense multi-modal transformers for handling text, images, and speech demands substantial computational resources, which limits their scalability and accessibility. An efficient architecture is required to reduce floating-point operations (FLOPs) while maintaining high performance across modalities.
How?: The research introduces the Mixture-of-Transformers (MoT), a sparse multi-modal transformer architecture. MoT separates model parameters by modality while maintaining global self-attention, thus allowing modality-specific processing. This separation reduces the computational cost by utilizing fewer floating-point operations (FLOPs) than dense models.
Results: Research showcase a comparable performance to dense multi-modal models while using 55.8% fewer FLOPs, demonstrating significant resource savings.
Advanced Pruning, Layer Slicing, and Attention Mechanisms
Why?: Pruning LLMs can reduce their size and computational cost but often results in uneven performance across domains. This research addresses these imbalances by introducing a pruning method that maintains robust performance across diverse datasets and tasks.
How?: The research introduces DRPruning, integrating distributionally robust optimization to improve structured pruning. This method refines pruning and pretraining processes, automatically finding optimal reference losses and data ratios to prevent biased performance across different domains.
Results: Experiments in both monolingual and multilingual settings indicate DRPruning outperforms similarly sized models in pruning metrics such as perplexity, downstream tasks, and instruction tuning, enhancing robustness against distribution shifts.

Why?: A small fraction of LLM parameters, termed "super weights," are disproportionately critical for model performance. Understanding these parameters enables more efficient pruning and quantization while preserving accuracy.
How?: The study identifies 'super weights' using a data-free approach involving a single forward pass through the model. The impact of pruning these weights is examined by evaluating changes in perplexity and zero-shot accuracy. The researchers also examine how preserving 'super activations' affects quantization strategies.
Results: Pruning a single 'super weight' dramatically increases perplexity and reduces accuracy. Preserving these weights allows simple quantization methods to match state-of-the-art performance and enables larger quantization block sizes.

Why?: Transformers face quadratic complexity in their attention mechanism, making inference on long sequences computationally inefficient. Star Attention addresses this by reducing memory and compute requirements.
How?: A two-phase block-sparse approximation called Star Attention is introduced. Phase one uses blockwise-local attention processed in parallel across multiple hosts. Phase two applies sequence-global attention for query and response tokens attending to cached tokens. This method integrates seamlessly with global attention-trained LLMs.
Results: The method reduces memory requirements and inference times by up to 11x while maintaining 95-100% accuracy.

Why?: Transformer attention mechanisms suffer from poor gradient signal backpropagation, which can hinder learning and slow convergence. LASER addresses this issue by improving gradient flow in the attention mechanism.
How?: The researchers propose LASER, an attention mechanism with larger gradient signals than standard attention, implemented with minor modifications to existing setups. They conducted experiments with autoregressive LLMs up to 2.2 billion parameters.
Survey papers
Creative ways to use LLMs!!
SIMS: Simulating Human-Scene Interactions with Real World Script Planning - Generate scripts for human-scene interactions in physics-based animations, enabling more dynamic and realistic character motion for games and simulations.
AIDetx: a compression-based method for identification of machine-learning generated text - AIDetx leverages data compression techniques to accurately distinguish between human-written and AI-generated text, achieving exceptional detection accuracy with minimal computational cost.
Build An Influential Bot In Social Media Simulations With Large Language Models - A novel framework integrates LLMs into agent-based social media simulations, replicating opinion dynamics and influencer behavior to better understand public opinion formation.
CoVis: A Collaborative Framework for Fine-grained Graphic Visual Understanding - CoVis combines segmentation networks with LLM-based content generation to provide detailed and holistic graphic visual interpretations, improving the accessibility of complex visual data.
Automated Test Transfer Across Android Apps Using Large Language Models - LLMigrate simplifies and accelerates UI test transfer between Android apps, significantly reducing the time and effort required for mobile app testing.

PanoLlama transforms panoramic image generation by reimagining it as a next-token prediction task, enabling endless, coherent panoramas with enhanced scalability and precision.
From CISC to RISC: language-model guided assembly transpilation - CRT automates the translation of x86 assembly code to ARM, facilitating the transition to more energy-efficient architectures while ensuring high accuracy and performance gains.
Multiverse of Greatness: Generating Story Branches with LLMs - Generate branching storylines with enhanced coherence and creativity, revolutionizing AI-driven storytelling for visual novels and dynamic narratives.
Reply