- LLMs Research
- Posts
- Meta Llama3 toped the leaderboard!
Meta Llama3 toped the leaderboard!
Large language models and related research papers published on April 18th 2024
New Models & π₯ research:
Meta published Llama 3! π¦
Total 3 papers published (discussed below) who tried increasing the context length of LLMs!!
Llama 3
Meta published Llama 3 yesterday and here is a quick summary:
8B and 70B models launched
8k context length
These models became the fastest models to claim a top position on HuggingFace leaderboard
They were trained on a dataset 7x larger than Llama 2 with 15T tokens
Llama3 can be accessed at meta.ai site and it will also be integrated on all offerings of Meta e.g. Facebook, messenger, etc.
TriForce: Lossless Acceleration of Long Sequence Generation with Hierarchical Speculative Decoding π₯π₯π₯

The key-value (KV) cache grows linearly in size with the sequence length.
The research paper proposes a solution called TriForce, which is a hierarchical speculative decoding system. It leverages the original model weights and dynamic sparse KV cache to create a draft model as an intermediate layer in the hierarchy. This draft model is then further speculated by a smaller model to reduce drafting latency. This approach allows for impressive speedups and scalability in handling even longer contexts, without compromising on the generation quality.
πResults:
The research paper achieves significant performance improvements with TriForce. On an A100 GPU, it achieves up to 2.31 times speedup for Llama2-7B-128K and only half the latency of the auto-regressive baseline on an A100 for the offloading setting on two RTX 4090 GPUs, with a speedup of 7.78 times on the optimized offloading system. Additionally, it outperforms DeepSpeed-Zero-Inference by 4.86 times on a single RTX 4090 GPU.

π€Problem?:
The research paper try to align pre-trained LLMs with human values and intentions.
π»Proposed solution:
The research paper proposes a new approach called Token-level Direct Preference Optimization (TDPO) to solve this problem. TDPO works by optimizing policy at the token level, incorporating forward KL divergence constraints for each token. This improves alignment and diversity, while also utilizing the Bradley-Terry model for a token-based reward system. Unlike previous methods, TDPO does not require explicit reward modeling, making it simpler and more efficient.
πResults:
The research paper achieved significant performance improvements in various text tasks. It strikes a better balance between alignment and generation diversity compared to other methods, particularly in controlled sentiment generation and single-turn dialogue datasets. Additionally, it significantly improves the quality of generated responses compared to other reinforcement learning-based methods.

Large number of parameters introduces significant latency in the LLMs inference.
π»Proposed solution:
The research paper proposes a novel parallel decoding approach called "hidden transfer" which allows for the simultaneous generation of multiple tokens in a single forward pass. This is achieved by transferring intermediate hidden states from the previous context to the "pseudo" hidden states of future tokens, which then pass through the following transformer layers to assimilate more semantic information and improve predictive accuracy.
This paper also introduces a tree attention mechanism to generate and verify multiple candidates of output sequences, ensuring lossless generation and further improving efficiency.
πGitHub: https://github.com/dwzhu-pku/LongEmbed
π€Problem?:
LLMs currently have limited context window size
π»Proposed solution:
The research paper proposes a training-free context window extension strategy, specifically using position interpolation, to effectively extend the context window of existing embedding models. This approach works by leveraging the existing model's ability to capture short contexts and extending it to longer contexts without the need for additional training. The paper also explores the use of specific techniques, such as NTK and SelfExtend, for models using rotary position embedding (RoPE) to further enhance performance.
πResults:
The research paper reports significant improvements in the performance of existing embedding models when extended to a larger context window of 32k tokens. These improvements are observed in both synthetic and real-world tasks, indicating the effectiveness of the proposed approach. Additionally, the paper also releases two new embedding models, E5-Base-4k and E5-RoPE-Base, along with a benchmark dataset, LongEmbed, to facilitate further research in this area.

π»Proposed solution:
The paper proposes a framework called multi-Agent Blockchain-inspired Collaboration (mABC) for root cause analysis in micro-services architecture. This framework utilizes multiple agents based on powerful language models to perform blockchain-inspired voting and reach a final agreement on the root cause of an issue. These agents are specialized and provide valuable insights based on their expertise and knowledge, collaborating within a decentralized chain. The decision-making process is inspired by blockchain governance principles and considers the contribution index and expertise index of each agent. The framework also includes an Agent Workflow, where tasks and queries are processed and distributed to the agents. This automated process greatly improves the efficiency and accuracy of root cause analysis in micro-services architecture.
πHuggingFace model: https://huggingface.co/collections/SurgeGlobal/open-bezoar-6620a24923e12127e9e2b9cc
Research paper proposes a recipe for fine-tuning LLMs using a combination of techniques.
First, they generate synthetic fine-tuning data using open and commercially non-restrictive variants of existing LLM models.
Then, they filter this data using GPT-4 as a human proxy.
Next, they use a cost-effective supervised fine-tuning method called QLoRA (Quality Loss over Randomized Alignments) with three different schemes.
The resulting checkpoint is further fine-tuned using a subset of the HH-RLHF dataset to minimize distribution shift
The final checkpoint is obtained using the DPO (Distributional Policy Optimization) loss. This approach aims to improve the performance of fine-tuned LLMs while also reducing their parameter count.
De-DSI: Decentralised Differentiable Search Index
The research paper proposes a novel framework called De-DSI, which combines large language models (LLMs) with genuine decentralization. This is achieved through the use of differentiable search index (DSI) concept, which operates solely on query-docid pairs. To enhance scalability, an ensemble of DSI models is used, where the dataset is partitioned into smaller shards for individual model training. This not only maintains accuracy but also facilitates scalability by aggregating outcomes from multiple models. The aggregation process uses a beam search to identify top docids and applies a softmax function for score normalization, selecting documents with the highest scores for retrieval. This decentralized implementation allows for the retrieval of multimedia items through magnet links, eliminating the need for intermediaries or platforms.

The research paper proposes AlphaLLM, a self-improvement method that integrates Monte Carlo Tree Search (MCTS)with LLMs. This creates a self-improving loop, allowing LLMs to refine their outputs and learn from self-assessed rewards. AlphaLLM also includes a prompt synthesis component, an efficient MCTS approach tailored for language tasks, and a trio of critic models for precise feedback. It works by addressing the unique challenges of combining MCTS with LLMs, such as data scarcity, vast search spaces, and subjective feedback in language tasks.
πResults:
The research paper shows that AlphaLLM significantly enhances the performance of LLMs in mathematical reasoning tasks without additional annotations, demonstrating the potential for self-improvement in LLMs. Thus, it achieves a performance improvement in LLMs' reasoning abilities.

The paper introduces a novel video summarization framework, V2Xum-LLM, which unifies different video summarization tasks into one large language model's (LLM) text decoder and achieves task-controllable video summarization through temporal prompts and task instructions.
The research paper proposes a new cross-modal video summarization dataset, Instruct-V2Xum, featuring 30,000 diverse videos sourced from YouTube with paired textual summaries referencing specific frame indexes.
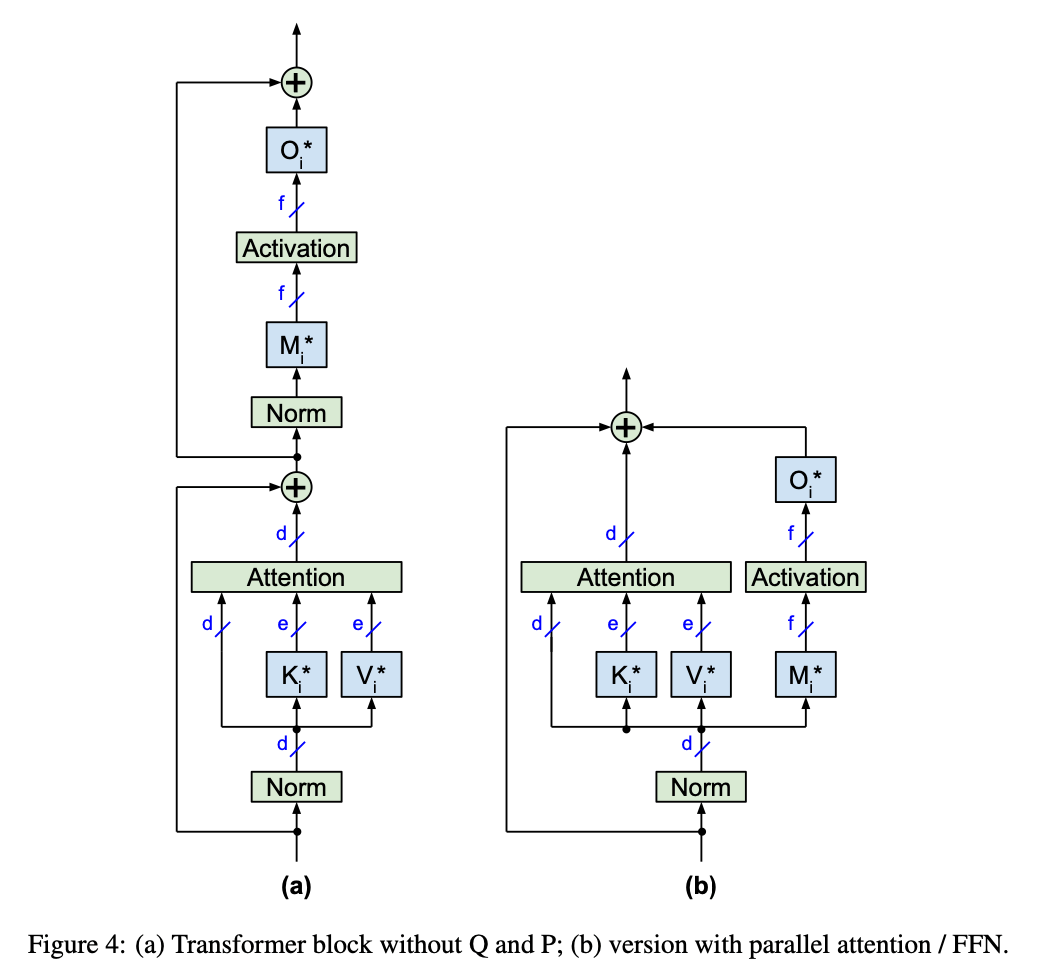
πGitHub: https://github.com/OpenMachine-ai/transformer-tricks
The research paper addresses the issue of high computational and memory complexity in large language models (LLMs) due to the use of post-attention projection (P) and linear layers.
The research paper proposes a skipless transformer without the V and P linear layers, which reduces the total number of weights and thus decreases compute and memory complexity.
This is achieved by removing the Q and P layers from the skipless version of popular LLMs such as Mistral-7B, which is suitable for multi-query attention (MQA) and grouped-query attention (GQA). This is mathematically equivalent to the original architecture but results in a significant reduction in weights. The skipless transformer works by directly using the output of multi-head attention (MHA) without the need for additional linear layers.
Database/benchmarks/ Evaluation framework:
πWant to learn more, Survey paper:
π§―Letβs make LLMs safe!! (LLMs security related papers)
π Creative ways to use LLMs!! (Applications based papers)
Reply