- LLMs Research
- Posts
- Summary of top LLMs related research papers published on April 4th, 2024
Summary of top LLMs related research papers published on April 4th, 2024
Simple explanation of important research papers published on April 4th for LLMs
Dear subscribers,
I mark recommended read(best papers) with “🔥” emoji so that you don’t miss important research papers. Just like how you missed “Mixture-of-Depths: Dynamically allocating compute in transformer-based language models” paper by Google DeepMind which I covered early and you missed it 😄 This paper is catching a lot of traction so from now onwards, don’t miss papers marked with 🔥
New Models:
Sailor: Open Language Models for South-East Asia - New model for South-East Asian language (Chinese, Vietnamese, Thai, Indonesian, Malay, and Lao)
AutoWebGLM: Bootstrap And Reinforce A Large Language Model-based Web Navigating Agent [Must read paper 🔥🔥]

🔗Code/data/weights:https://github.com/THUDM/AutoWebGLM
🤔Problem?:
The research paper addresses the problem of unsatisfactory performance of existing web navigation agents due to the versatility of actions on webpages, limitations in processing HTML text, and the complexity of decision-making in an open-domain web environment.
💻Proposed solution:
The research paper proposes a solution called AutoWebGLM, which is an automated web navigation agent built upon ChatGLM3-6B and inspired by human browsing patterns. It uses an HTML simplification algorithm to represent webpages in a concise manner and employs a hybrid human-AI method to build web browsing data for curriculum training. The model is then bootstrapped using reinforcement learning and rejection sampling, allowing it to comprehend webpages, perform browser operations, and efficiently decompose tasks.
Training LLMs over Neurally Compressed Text [Must read 🔥] [Google DeepMind & Anthropic AI]

🤔Problem?:
The research paper addresses the problem of training large language models (LLMs) over highly compressed text. This is important because standard subword tokenizers can only achieve a small level of compression, while neural text compressors can achieve much higher rates of compression. This presents an opportunity for more efficient training and serving of LLMs, as well as easier handling of long text spans. However, the main obstacle to this goal is that strong compression tends to produce opaque outputs that are not well-suited for learning.
💻Proposed solution:
To solve this problem, the research paper proposes a novel compression technique called Equal-Info Windows. This method involves segmenting the text into blocks that each compress to the same bit length. This allows for effective learning over neurally compressed text that improves with scale. The method outperforms byte-level baselines on perplexity and inference speed benchmarks. While it may have worse perplexity than subword tokenizers for models trained with the same parameter count, it has the benefit of shorter sequence lengths. This reduces the number of autoregressive generation steps and decreases latency.
📊Results:
The research paper does not explicitly mention any performance improvement achieved, but it does state that the proposed method outperforms byte-level baselines on
Unveiling LLMs: The Evolution of Latent Representations in a Temporal Knowledge Graph [very interesting paper - Again must read 🔥]
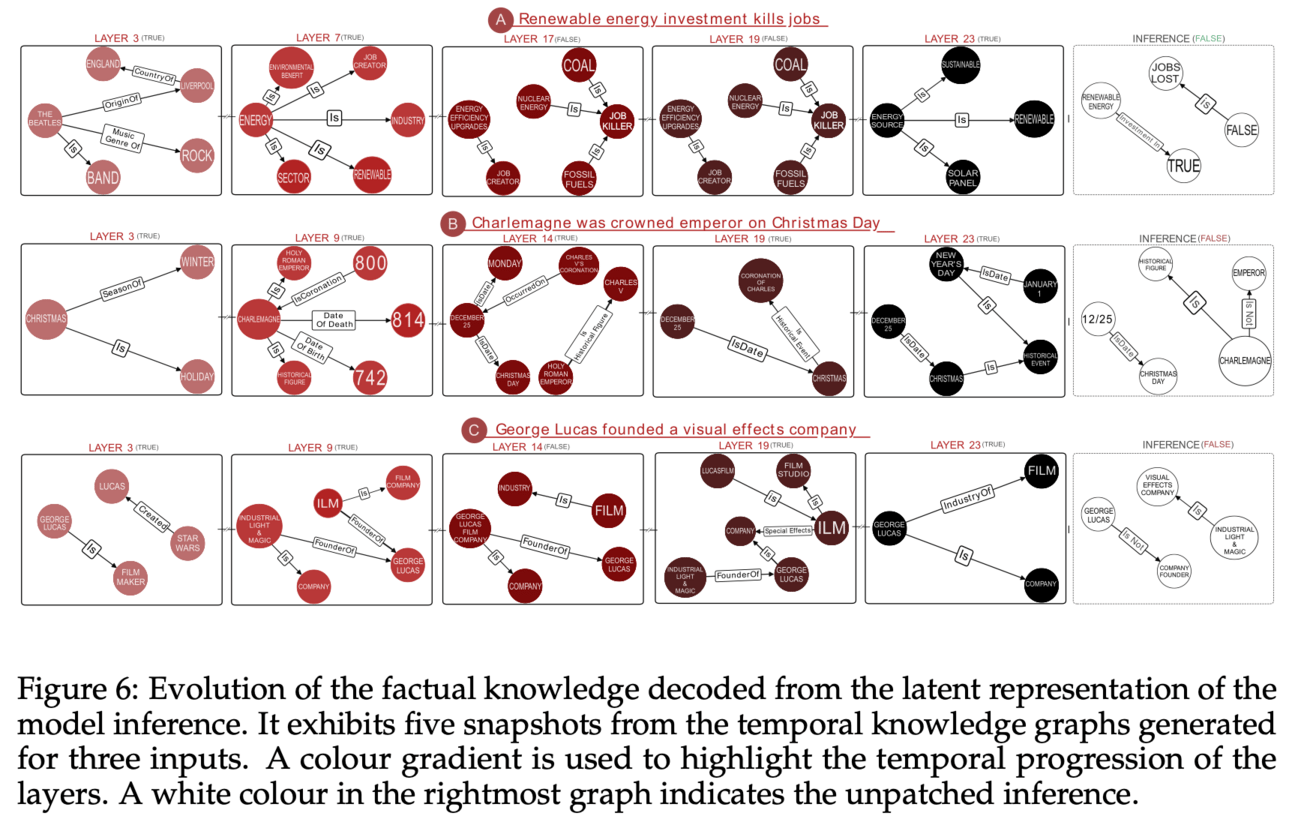
🤔Problem?: This research paper addresses the problem of understanding and explaining the internal mechanisms of Large Language Models (LLMs) in order to better interpret their behavior and decision-making process.
💻Proposed solution: It proposes an end-to-end framework that decodes the factual knowledge embedded in LLMs' latent space by converting it from a vector space to a set of ground predicates and representing its evolution across layers using a temporal knowledge graph. This framework uses the technique of activation patching, which dynamically alters the latent representations of the model during inference. This approach does not require external models or training processes, making it more efficient and effective in providing interpretability.
Visualization-of-Thought Elicits Spatial Reasoning in Large Language Models [Microsoft Research] 🔥

🤔Problem?: The research paper addresses the relatively unexplored ability of large language models (LLMs) in spatial reasoning, which is a crucial aspect of human cognition.
💻Proposed solution: The research paper proposes a method called Visualization-of-Thought (VoT) prompting to enhance the spatial reasoning abilities of LLMs. VoT works by visualizing the reasoning traces of LLMs, thereby guiding their subsequent reasoning steps. It is inspired by the cognitive capacity of human beings to create mental images of unseen objects and actions, known as the Mind's Eye.
📊Results:
The research paper demonstrated that VoT significantly improves the spatial reasoning abilities of LLMs, outperforming existing multimodal large language models (MLLMs) in tasks such as natural language navigation, visual navigation, and visual tiling in 2D grid worlds. This suggests the potential viability of VoT in MLLMs as well, as it mimics the mind's eye process of generating mental images to facilitate spatial reasoning.

🤔Problem?:
The research paper addresses the problem of video understanding, specifically the challenge of processing and comprehending both visual and textual data in videos.
💻Proposed solution:
The paper proposes a multimodal Large Language Model (LLM) called MiniGPT4-Video, which is designed to process both visual and textual data in a sequence of frames. This is achieved by building upon the success of MiniGPT-v2, which was able to translate visual features into the LLM space for single images. MiniGPT4-Video extends this capability to videos by incorporating both visual and text components, allowing it to effectively answer queries involving both modalities.
📊Results:
The proposed model outperforms existing state-of-the-art methods on multiple benchmarks, including the MSVD, MSRVTT, TGIF, and TVQA datasets. The model achieved gains of 4.22%, 1.13%, 20.82%, and 1

The research paper addresses the potential limitations of existing memory-augmented methods for personalized response generation using large language models (LLMs). The research paper proposes a novel approach called Memory-Injected approach using Parameter-Efficient Fine-Tuning (PEFT) along with a Bayesian Optimization searching strategy, to achieve LLM Personalization (MiLP). This approach aims to address the limitations of existing methods by injecting user-specific knowledge into the LLM model during fine-tuning, instead of relying solely on pre-stored knowledge. This allows for a more personalized and fine-grained understanding of user queries, leading to more accurate and relevant responses.

🤔Problem?:
The research paper addresses the problem of limited video summarization datasets, which hinders the effectiveness of state-of-the-art methods for generalization.
💻Proposed solution:
The research paper proposes a solution by using large language models (LLMs) as Oracle summarizers to generate a large-scale video summarization dataset. This dataset is created by leveraging the abundance of long-form videos with dense speech-to-video alignment. The proposed approach also includes a new video summarization model that effectively addresses the limitations of existing approaches. It works by using LLMs to summarize long text and generate high-quality summaries for each video in the dataset.
📊Results:
The research paper achieved significant performance improvement, as indicated by the extensive experiments conducted. It sets a new state-of-the-art in video summarization across several benchmarks, showcasing the effectiveness of the proposed approach. Additionally, the paper also presents a new benchmark dataset containing 1200 long videos with high-quality summaries annotated by professionals, which can further facilitate research in the field.

🔗Code/data/weights:https://github.com/ziplab/LongVLM
🤔Problem?:
The research paper addresses the challenges faced by existing VideoLLMs in achieving detailed understanding in long-term videos due to overlooking local information.
💻Proposed solution:
The research paper proposes a straightforward yet powerful VideoLLM called LongVLM that decomposes long videos into multiple short-term segments and encodes local features for each segment using a hierarchical token merging module. These features are then concatenated in temporal order to maintain the storyline across sequential short-term segments. Additionally, global semantics are integrated into each local feature to enhance context understanding. This way, the VideoLLM can generate comprehensive responses for long-term videos by incorporating both local and global information.
📊Results:
The research paper demonstrates the superior capabilities of LongVLM over previous state-of-the-art methods through experiments on the VideoChatGPT benchmark and zero-shot video question-answering datasets. Qualitative examples also show that LongVLM produces more precise responses for long video understanding.

The paper proposes a method called eigenpruning, which involves removing singular values from weight matrices in an LLM. This is inspired by interpretability methods that automatically find subnetworks within a model that can effectively solve a given task. By pruning the weight matrices, the model becomes more efficient in solving the task at hand.
Papers with database/benchmarks:
Capabilities of Large Language Models in Control Engineering: A Benchmark Study on GPT-4, Claude 3 Opus, and Gemini 1.0 Ultra - Benchmark to test the breadth, depth, and complexity of classical control design using LLMs.
ReaLMistake A detailed benchmark to test LLMs in 4 core categories - GitHub
Intent Detection and Entity Extraction from BioMedical Literature
Untangle the KNOT: Interweaving Conflicting Knowledge and Reasoning Skills in Large Language Models
CodeEditorBench: Evaluating Code Editing Capability of Large Language Models
Evaluating Generative Language Models in Information Extraction as Subjective Question Correction
Towards Pareto Optimal Throughput in Small Language Model Serving
How Easily do Irrelevant Inputs Skew the Responses of Large Language Models?
Testing the Effect of Code Documentation on Large Language Model Code Understanding
📚Want to learn more, Survey paper:
🧯Let’s make LLMs safe!! (LLMs security related papers)
Learn When (not) to Trust Language Models: A Privacy-Centric Adaptive Model-Aware Approach
Red Teaming GPT-4V: Are GPT-4V Safe Against Uni/Multi-Modal Jailbreak Attacks?
Towards Standards-Compliant Assistive Technology Product Specifications via LLMs
The Artificial Intelligence Ontology: LLM-assisted construction of AI concept hierarchies
🌈 Creative ways to use LLMs!! (Applications based papers)
Reply