- LLMs Research
- Posts
- Summary of top LLMs related research papers published on April 8th, 2024
Summary of top LLMs related research papers published on April 8th, 2024
Simple explanation of important research papers published on April 8th for LLMs
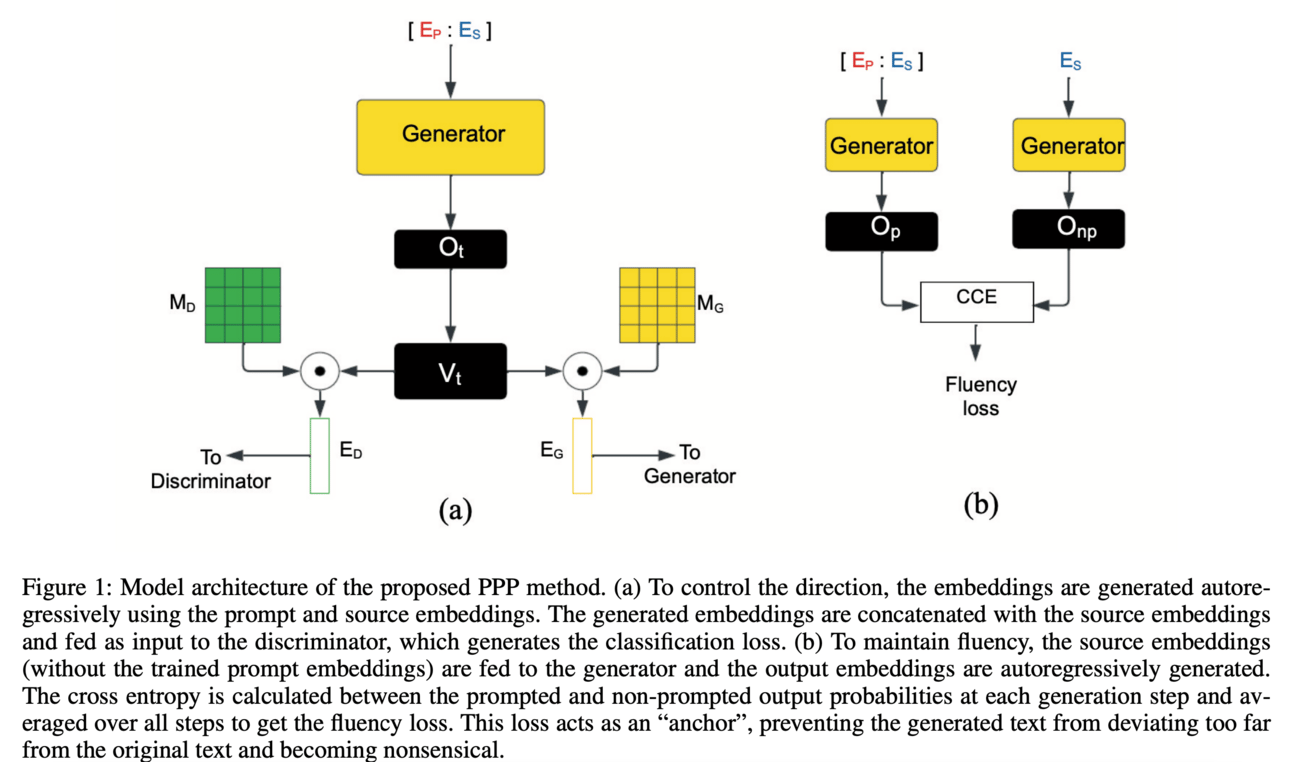
π€ Problem?: The research paper addresses the challenge of controlling the direction of language generation using textual prompts with smaller language models.
π» Proposed solution: The paper proposes a method called Prompt Tuning, which uses prompt embeddings trained with a small language model to steer the generated text. The prompt embeddings act as a discriminator, allowing for controlled language generation. The embeddings can be trained with a very small dataset, making the method data and parameter efficient.
π Results: The research paper demonstrates the efficacy of their method through extensive evaluation on four datasets, achieving improvements in sentiment analysis, formality, and mitigating harmful and toxic language generated by language models.

π€Problem?:
The research paper addresses the problem of enhancing the performance of large language models (LLM) on downstream tasks, while also maintaining privacy protection for user data.
π»Proposed solution:
The research paper proposes a distributed PEFT framework called DLoRA, which enables collaborative fine-tuning operations between the cloud and user devices. This framework utilizes a Kill and Revive algorithm to reduce computation and communication workload over user devices while still achieving high accuracy and privacy protection.
πResults:
The research paper shows that DLoRA significantly reduces computation and communication workload over user devices while also achieving superior accuracy and privacy protection. This performance improvement is achieved through the use of the proposed Kill and Revive algorithm in the distributed PEFT framework.

π€Problem?:
The research paper addresses the problem of document understanding, specifically the lack of utilization of document layout information in previous methods that employ large language models (LLMs) or multimodal large language models (MLLMs).
π»Proposed solution:
The research paper proposes a method called LayoutLLM, which is based on LLMs/MLLMs and utilizes a layout instruction tuning strategy to enhance the comprehension and utilization of document layouts. This strategy consists of two components: Layout-aware Pre-training and Layout-aware Supervised Fine-tuning. In Layout-aware Pre-training, three groups of pre-training tasks are introduced to capture document-level, region-level, and segment-level information. Additionally, a module called layout chain-of-thought (LayoutCoT) is devised to help LayoutLLM focus on regions relevant to the question and generate accurate answers. This module also improves the interpretability of the results, making it easier for manual inspection and correction.

π€Problem?:
The research paper aims to address the issue of semantic drift in modern language models (LLMs), which tend to generate correct facts initially but gradually drift away and generate incorrect facts as they continue to generate text. This issue has been observed before, but has never been properly measured or addressed.
π»Proposed solution:
The research paper proposes to solve this problem by developing a semantic drift score, which measures the degree of separation between correct and incorrect facts in the generated text. This score is used to identify when the model is starting to generate incorrect facts and thus, early stopping methods can be applied to improve factual accuracy. Additionally, the paper suggests using semantic similarity reranking as a further improvement to the baseline method. This involves comparing the generated text with a reference text and re-ranking it based on semantic similarity. The paper also explores the use of external APIs to bring the model back to the right generation path, but does not report positive results.

π»Proposed solution:
The research paper proposes an automated approach, called AutoCodeRover, which combines Large Language Models (LLMs) with sophisticated code search capabilities to autonomously solve Github issues and achieve program improvement. It works by using LLM-based programming assistants to modify or patch code, while also utilizing program structure and spectrum-based fault localization to enhance understanding of the issue's root cause and retrieve a context via iterative search.
πResults: The research paper shows increased efficacy in resolving more than 20% of real-life Github issues involving bug fixing and feature additions, as compared to recent efforts from the AI community. This suggests a significant performance improvement in automating the software development process.

π€Problem?:
The research paper addresses the problem of handling a broad range of multimodal problems in real-world AI systems, specifically in the context of Visual Question Answering (VQA).
π»Proposed solution:
The research paper proposes a Hierarchical MultiModal React (HAMMR) approach, which builds upon the existing paradigm of combining Large Language Models (LLMs) with external specialized tools. HAMMR enables the LLM+tools approach to handle a varied suite of VQA tasks by making it hierarchical and allowing specialized agents to be called upon. This enhances the compositionality of the approach and leads to better results on generic VQA tasks.
πResults:
The research paper reports a performance improvement of 19.5% on a generic VQA suite when compared to the naive LLM+tools approach. Additionally, HAMMR achieves state-of-the-art results on the task, outperforming the generic standalone PaLI-X VQA model by 5.0%.

πCode/data/weights:https://github.com/JacobChalk/TIM
π€Problem?:
The research paper addresses the problem of recognizing and detecting actions in long videos using both audio and visual signals. It aims to improve the performance of existing methods by explicitly modeling the temporal extents of audio and visual events and their interaction.
π»Proposed solution:
The research paper proposes the Time Interval Machine (TIM) which uses a transformer encoder to process long videos based on modality-specific time intervals. These intervals serve as queries for the encoder to attend to, along with the surrounding context in both modalities. By integrating the two modalities and explicitly modeling their time intervals, TIM is able to recognize and detect actions more accurately.
πResults:
The research paper reports state-of-the-art results on three long audio-visual video datasets: EPIC-KITCHENS, Perception Test, and AVE. On EPIC-KITCHENS, TIM outperforms previous methods that utilize larger pre-training by 2.9% in top-1 action recognition accuracy. It also shows strong performance on the Perception Test and outperforms other methods on EPIC-KITCHENS-100 for most metrics when adapted for action detection. These results demonstrate the effectiveness of TIM in improving the performance of action recognition and detection in long videos.

π€Problem?:
The research paper addresses the issue of computational and parameter efficiency in Mixture-of-Experts (MoE) language models. These models have shown promise in reducing computational costs compared to dense models, but at the expense of requiring more parameters. This makes them less efficient in certain scenarios, such as autoregressive generation, where large GPU memory requirements are a limiting factor.
π»Proposed solution:
The research paper proposes a hybrid dense training and sparse inference framework for MoE models, called DS-MoE. This framework utilizes dense computation during training and sparse computation during inference, allowing for strong computation and parameter efficiency. During training, all experts in the MoE model are activated, while during inference, only a subset of experts are activated based on the input. This results in a more efficient use of parameters and computational resources.
πResults:
The research paper reports that their DS-MoE models are more parameter-efficient than standard sparse MoEs and perform on par with dense models in terms of total parameter size and performance. In addition, their DS-MoE-6B model runs up to 1.86 times faster than similar dense models and between 1.50 and 1.71 times faster than comparable MoEs. These results demonstrate the effectiveness

πGitHub: https://boheumd.github.io/MA-LMM/.
π€Problem?:
The research paper addresses the issue of limited frame processing in video understanding for large language models (LLMs). Existing LLM-based multimodal models can only take in a small number of frames, which hinders their ability to analyze long-term video content.
π»Proposed solution:
To solve this problem, the research paper proposes a new model that processes videos in an online manner and stores past video information in a memory bank. This allows the model to reference historical video content for long-term analysis without exceeding LLMs' context length constraints or GPU memory limits. The memory bank can be seamlessly integrated into existing multimodal LLMs, making it an efficient and effective solution for long-term video understanding.
πResults:
The research paper conducted extensive experiments on various video understanding tasks, such as long-video understanding, video question answering, and video captioning. The proposed model achieved state-of-the-art performances across multiple datasets, showcasing its effectiveness in addressing the problem of limited frame processing in LLM-based multimodal models. The code for the model is also available for further usage and improvement.
Papers with database/benchmarks:
πWant to learn more, Survey/Review paper:
π§―Letβs make LLMs safe!! (LLMs security related papers)
π Creative ways to use LLMs!! (Applications based papers)
Reply