- LLMs Research
- Posts
- Summary of top LLMs related research papers published on March 25th, 2024
Summary of top LLMs related research papers published on March 25th, 2024

Referring Single Object Tracking (RSOT) of Elysium
π Project demo: https://github.com/Hon-Wong/Elysium
π€Problem?:
The research paper aims to explore the use of Multi-modal Large Language Models (MLLMs) in video-related tasks, specifically in object tracking. It addresses the lack of exploration in this area and highlights two key challenges that hinder the application of MLLMs in video tasks.
π»Proposed solution:
To solve the problem, the research paper introduces ElysiumTrack-1M, a large-scale video dataset with annotated frames, object boxes, and descriptions. It also proposes two novel tasks, Referring Single Object Tracking (RSOT) and Video Referring Expression Generation (Video-REG), to train MLLMs on this dataset. Additionally, the paper introduces a token-compression model called T-Selector to handle the computational burden of processing a large number of frames within the context window of Large Language Models (LLMs).
πResults:
The research paper does not provide any specific performance improvement achieved by their proposed approach, Elysium. However, it is the first attempt to conduct object-level tasks in videos using MLLMs without the need for any additional plug-ins or expert models. This showcases the potential and effectiveness of using MLLMs in video tasks.
π»Proposed solution:
Paper proposes a ProCoder, which aims to iteratively refine the project-level code context for precise code generation. This is done by leveraging compiler feedback to identify mismatches between the generated code and the project's context. ProCoder then aligns and fixes these errors using information extracted from the code repository. This iterative process allows the model to explore and incorporate project-specific context that cannot fit into LLM prompts, resulting in more accurate and reliable code generation.
πResults:
The research paper reports a significant improvement in the performance of vanilla LLMs, by over 80%, in generating code that is dependent on project context.

Architecture of DOrA
π€Problem?:
Paper addresses the problem of 3D visual grounding, specifically in the context of identifying a target object within a 3D point cloud scene based on a natural language description.
π»Proposed solution:
The research paper proposes a novel 3D visual grounding framework called DOrA, which stands for Order-Aware referring. DOrA leverages Large Language Models (LLMs) to parse language descriptions and suggest a referential order of anchor objects. This order is important as it allows DOrA to update visual features and accurately locate the target object during the grounding process. This is achieved through a combination of cross-modal transformers and the use of ordered anchor objects.
πResults:
The research paper provides experimental results on two datasets, NR3D and ScanRefer, to demonstrate the performance of DOrA. In both low-resource and full-data scenarios, DOrA shows superiority over current state-of-the-art frameworks. Specifically, DOrA achieves a 9.3% and 7.8% improvement in grounding accuracy under 1% data and 10% data settings, respectively

π€Problem?:
The research paper addresses the potential for fully automated software development by introducing a new task called Natural Language to code Repository (NL2Repo). This task aims to generate an entire code repository from its natural language requirements.
π»Proposed solution:
The research paper proposes a framework called CodeS to solve the NL2Repo task. CodeS decomposes the task into multiple sub-tasks by using a multi-layer sketch. It includes three modules: RepoSketcher, FileSketcher, and SketchFiller. RepoSketcher generates the directory structure of the repository, FileSketcher generates a file sketch for each file, and SketchFiller fills in the details for each function in the file sketch. This framework is designed to be simple yet effective in generating code repositories from natural language requirements.
πResults:
The research paper provides evidence of the effectiveness and practicality of CodeS through extensive experiments. It showcases the performance improvement of CodeS through both automated benchmarking and manual feedback analysis. The benchmark-based evaluation uses a repository-oriented benchmark, SketchEval, and an evaluation metric called SketchBLEU. The feedback-based evaluation involves engaging 30 participants in empirical studies using a VSCode plugin for CodeS. The results of these experiments prove the effectiveness and practicality of Code.
Can LLMs replace one pilot in βοΈ?π

Dear readers,
Letβs take a pause for a second! If you want to gain a proficiency in LLMs or in search of a book for a weekend read then here are my recommendations!!
Natural Language Processing with Transformers, Revised Edition - Lewis Tunstall, Leandro von Werra, Thomas Wolf
Generative Deep Learning: Teaching Machines to Paint, Write, Compose, and Play - David Foster, Karl Friston
Applied Machine Learning and AI for Engineers: Solve Business Problems That Can't Be Solved Algorithmically - Jeff Prosise


πCode/data/weights:https://github.com/MiuLab/InstUPR
π€Problem?:
The research paper addresses the problem of unsupervised passage reranking, specifically in the context of information retrieval. This is a challenging task as it requires accurately ranking passages based on their relevance to a given query, without the use of any training data or retrieval-specific instructions.
π»Proposed solution:
The research paper proposes a method called InstUPR, which stands for Instruction-based Unsupervised Passage Reranking. This method utilizes large language models (LLMs) that have been specifically tuned for instruction-following capabilities. This means that the LLMs have been trained to accurately answer questions and follow instructions, making them suitable for the task of passage reranking. The method also introduces a soft score aggregation technique, which combines different scores from the LLMs to produce a final relevance score for each passage. In addition, pairwise reranking is employed to further improve the performance of the method.
πResults:
The research paper provides experimental results on the BEIR benchmark dataset, which shows that InstUPR outperforms both unsupervised baselines and an instruction-tuned reranker. This highlights the effectiveness and superiority of the proposed method. The open-sourced source code allows for reproducibility of the experiments and further improvements in performance.
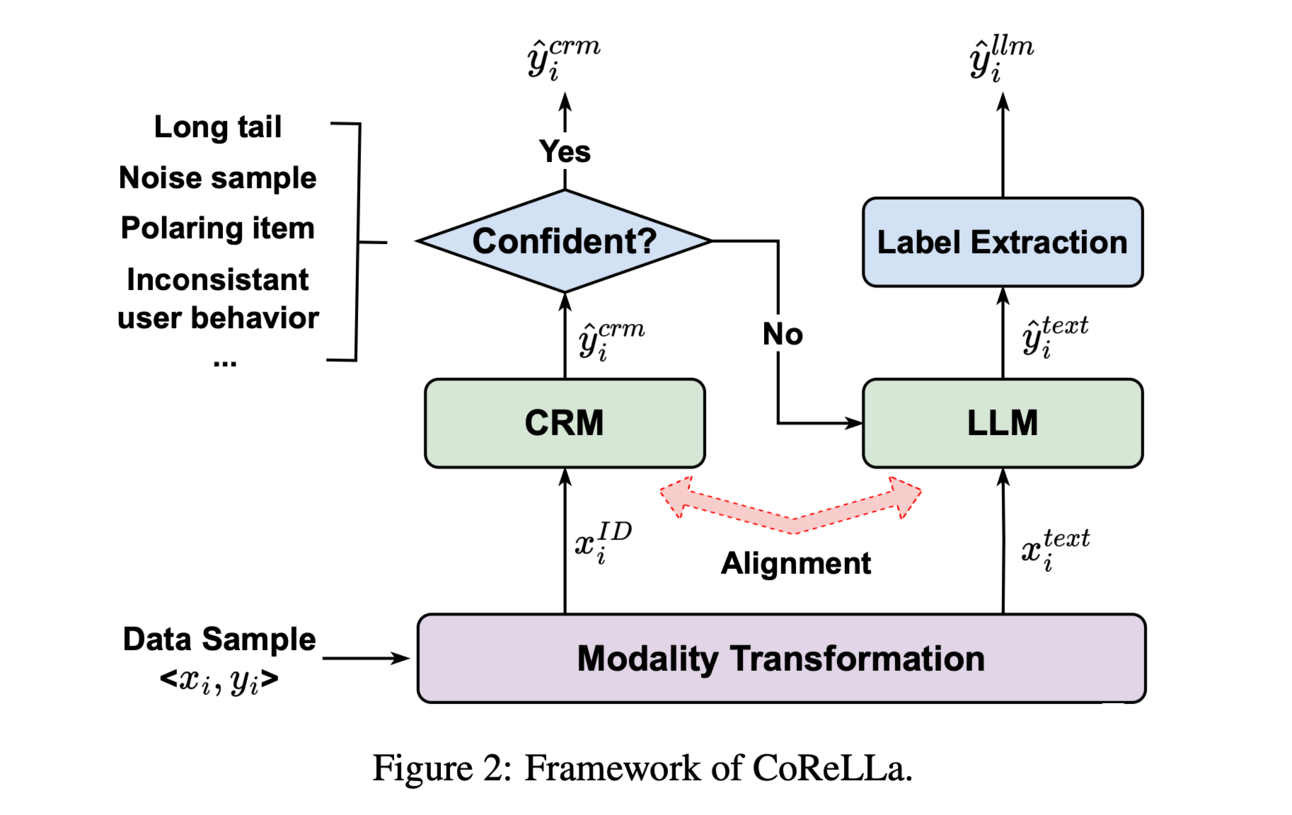
π» Proposed solution:
This research paper proposes a new framework called Collaborative Recommendation with conventional Recommender and Large Language Model (CoReLLa). This framework combines the strengths of both LLMs and CRMs by jointly training them and addressing the issue of decision boundary shifts through alignment loss. The resource-efficient CRM handles simple and moderate samples, while the LLM processes the small subset of challenging samples that the CRM struggles with.
πResults:
The research paper demonstrates that CoReLLa outperforms state-of-the-art CRM and LLM methods significantly in recommendation tasks. This improvement is achieved through the combined strengths of both LLM and CRM, leading to more accurate and efficient recommendations.

π€Problem?:
The research paper addresses the problem of debugging complex code, which can be time-consuming and challenging for programmers.
π»Proposed solution:
The paper proposes to solve this problem by introducing ChatDBG, an AI-powered debugging assistant. ChatDBG integrates large language models (LLMs) to enhance the capabilities and user-friendliness of conventional debuggers. It allows programmers to engage in a collaborative dialogue with the debugger, where they can ask complex questions about program state, perform root cause analysis, and explore open-ended queries. The LLM is granted autonomy to navigate through stacks and inspect program state, and then report its findings and yield back control to the programmer. This integration with standard debuggers such as LLDB, GDB, WinDBG, and Pdb for Python makes it easily accessible for programmers.
πResults:
The research paper demonstrates the effectiveness of ChatDBG through evaluation on a diverse set of code, including C/C++ code with known bugs and Python code. It shows that ChatDBG successfully analyzes root causes, explains bugs, and generates accurate fixes for a wide range of real-world errors. In the case of Python programs, a single query led to an actionable bug fix 67% of the time, and one additional follow-up query increased the success rate
Reply