- LLMs Research
- Posts
- Summary of top LLMs related research papers published on March 26th, 2024
Summary of top LLMs related research papers published on March 26th, 2024
Top research papers explained on large language models which were published on March 26th 2024
Dear reader,
I am blown away by seeing the quality of today’s research papers. I know you don’t have much time but don’t miss checking today’s papers. They are too good!! Lots of LLMs improvement techniques published today. Let’s start👇
[Highly recommended read & ridiculously detailed LLM model] 📚
This paper introduces InternLM2, an open-source LLM that outperforms its predecessors.
🔗 HuggingFace: https://huggingface.co/internlm/internlm2-20b
💻Proposed solution:
This model is meticulously pre-trained on diverse data types including text, code, and long-context data. This allows the model to efficiently capture long-term dependencies, leading to improved performance. Additionally, the paper discusses innovative pre-training and optimization techniques used in the development of InternLM.
📊Results:
The research paper reports a significant performance improvement of InternLM2 compared to its predecessors. It outperforms them in comprehensive evaluations across 6 dimensions and 30 benchmarks, long-context modeling, and open-ended subjective evaluations.

🤔Problem?:
The research paper addresses the issue of huge memory consumption in large language models (LLMs), which has become a major roadblock to large-scale training in the machine learning community. This is a problem because it limits the ability to train these models effectively and hinders their performance.
💻Proposed solution:
The research paper proposes a solution called Layer wise Importance Sampled AdamW (LISA), which aims to reduce the memory costs associated with training LLMs while still achieving high performance. LISA works by applying the concept of importance sampling to different layers in LLMs and randomly freezing most middle layers during optimization. This allows for more efficient use of memory resources and helps in achieving better performance.
📊Results:
The research paper reports significant performance improvements with LISA compared to other techniques such as Low-Rank Adaptation (LoRA) and full parameter training. In a range of settings, LISA outperforms LoRA and full parameter training by 11-37% in terms of MT-Bench scores. On large models, specifically LLaMA-2-70B, LISA achieves on-par or better performance than LoRA on various tasks such as MT-Bench, GSM8K, and PubMedQA, demonstrating its effectiveness across different domains

🤔Problem?:
The research paper addresses the issue of resolving emergent issues in GitHub repositories during software evolution. This is a complex challenge that involves both incorporating new code and maintaining existing functionalities.
💻Proposed solution:
The research paper proposes a novel LLM-based Multi-Agent framework for GitHub issue resolution, called MAGIS. This framework consists of four types of agents - Manager, Repository Custodian, Developer, and Quality Assurance Engineer - that work together to leverage the potential of LLMs in resolving GitHub issues. The framework utilizes collaboration and planning among the various agents to overcome the difficulties faced by LLMs in code change at the repository level.
📊Results:
Team employs the SWE-bench benchmark to compare MAGIS with popular LLMs, including GPT-3.5, GPT-4, and Claude-2. MAGIS can resolve 13.94% GitHub issues, which significantly outperforms the baselines.

🤔Problem?:
The research paper addresses the problem of reducing computational resources and improving the memory and latency of inference for large language models (LLMs).
💻Proposed solution:
The research paper proposes a simple layer-pruning strategy for popular families of open-weight pretrained LLMs. This involves identifying the optimal block of layers to prune based on similarity across layers, and then performing a small amount of finetuning using parameter-efficient finetuning (PEFT) methods such as quantization and Low Rank Adapters (QLoRA). This allows for the removal of up to half of the layers in the LLMs without significant performance degradation.
📊Results:
The research paper did not provide specific performance improvement results, but suggests that layer pruning methods can complement other PEFT strategies and improve the efficiency of finetuning and inference for LLMs.
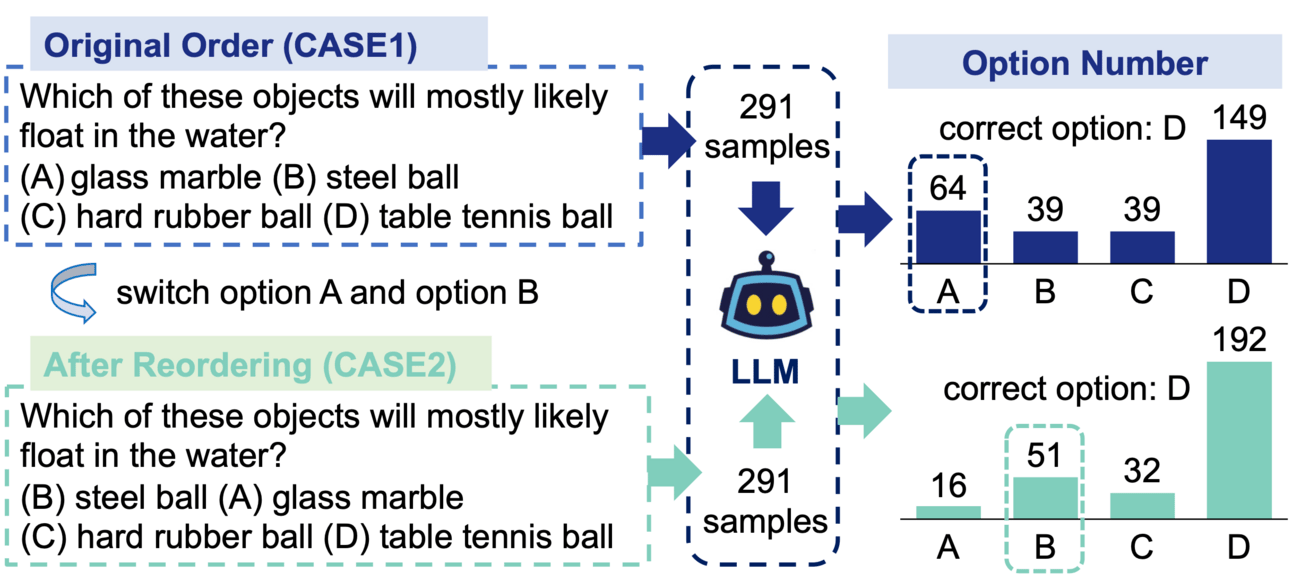
🔗Code/data/weights:https://github.com/Meetyou-AI-Lab/Can-MC-Evaluate-LLMs.
🤔Problem?:
The research paper addresses the issue of using multiple-choice questions (MCQs) as the primary method for evaluating large language models (LLMs). There are concerns that this method may not accurately measure the capabilities of LLMs, especially in knowledge-intensive scenarios where long-form generation (LFG) answers are required.
💻Proposed solution:
The research paper proposes to solve this problem by conducting a thorough analysis of MCQs' effectiveness in evaluating LLMs. This is done by evaluating nine LLMs on four question-answering (QA) datasets in two languages: Chinese and English. The researchers also identify a significant issue with LLMs exhibiting an order sensitivity in bilingual MCQs, favouring answers located at specific positions. To address this, they compare the results of MCQs with long-form generation questions (LFGQs) by analyzing their direct outputs, token logits, and embeddings. Additionally, the paper proposes two methods for quantifying the consistency and confidence of LLMs' output, which can be applied to other QA evaluation benchmarks.
📊Results:
The paper does not mention any specific performance improvement achieved, as the focus is on identifying the misalignment between MCQs and LFGQs.

🔗Code/data/weights:https://github.com/amurtadha/NBCE-master
🤔Problem?:
The research paper addresses the problem of length limitations in transformer architecture, which hinders the ability of Large Language Models (LLMs) to effectively integrate supervision from a substantial number of demonstration examples in In-Context Learning (ICL) approaches.
💻Proposed solution:
The research paper proposes a novel framework called Naive Bayes-based Context Extension (NBCE) to enable existing LLMs to perform ICL with an increased number of demonstrations by significantly expanding their context size. This is achieved by initially splitting the context into equal-sized windows, selecting the most relevant window through a voting mechanism, and using Bayes' theorem to generate the test task. Unlike other methods, NBCE does not require fine-tuning or dependence on particular model architectures, making it more efficient.
📊Results:
The research paper's experimental results showed that NBCE substantially enhances performance, particularly as the number of demonstration examples increases. It consistently outperforms alternative methods, demonstrating the effectiveness of the proposed framework in addressing the problem of length limitations in transformer architecture. The NBCE code will also be made publicly accessible, allowing others to use and improve upon it.

🤔Problem?:
The research paper addresses the challenges of practical inference for large language models (LLMs) in natural language processing (NLP).
💻Proposed solution:
The research paper proposes a novel algorithm-system co-design solution called ALISA. On the algorithm level, ALISA prioritizes important tokens using a Sparse Window Attention (SWA) algorithm, which introduces high sparsity in attention layers and reduces the memory footprint of KV caching. On the system level, ALISA employs three-phase token-level dynamical scheduling to optimize the trade-off between caching and recomputation, maximizing overall performance in resource-constrained systems.
📊Results:
The research paper demonstrates that ALISA improves the throughput of baseline systems, such as FlexGen and vLLM, by up to 3X and 1.9X, respectively, in a single GPU-CPU system under varying workloads.
Connect with fellow researchers community on Twitter to discuss more about these papers at
Language specific LLMs applications/dataset/benchmark
Reply